The Machine Learning Pipeline on AWS
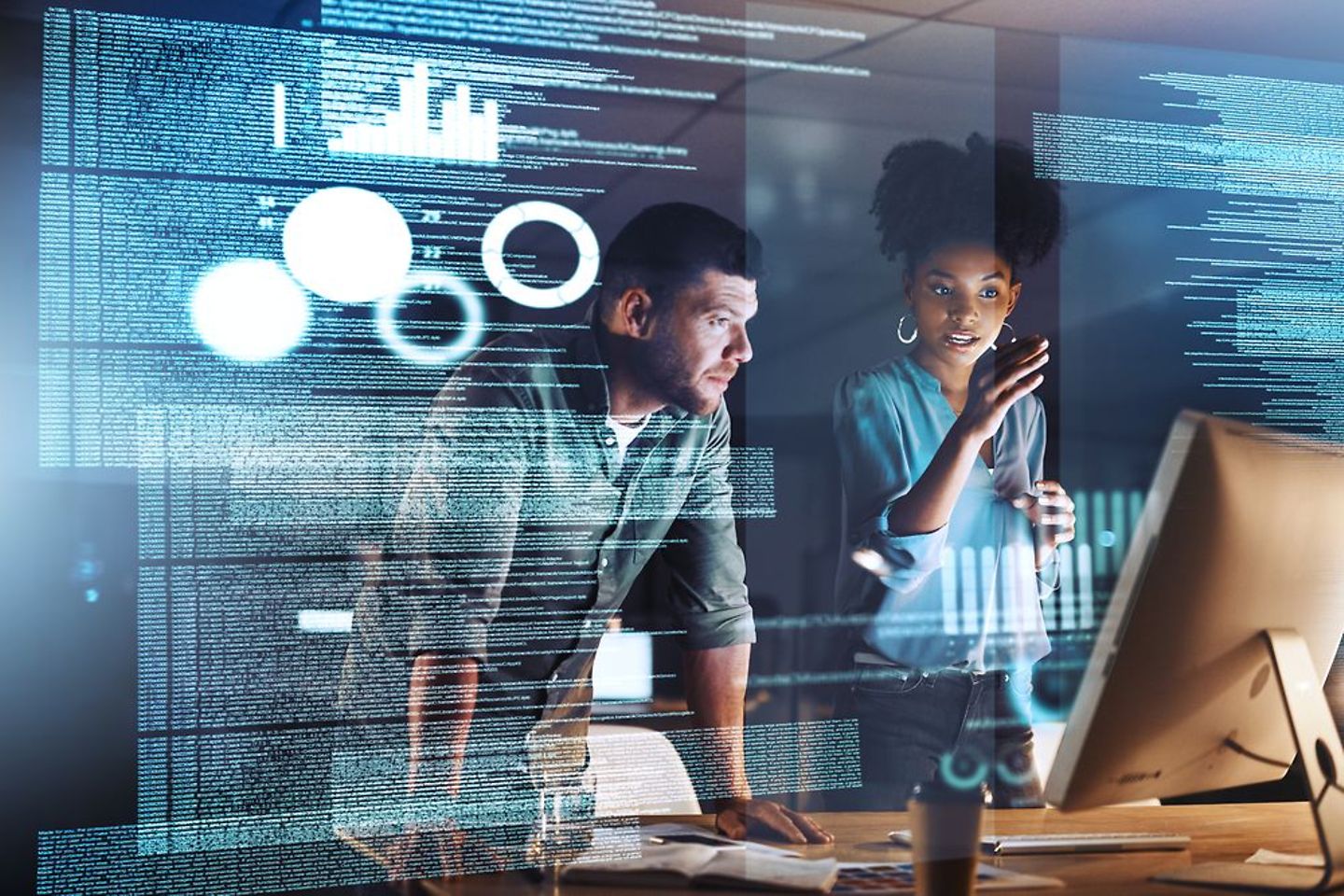
About Course
The Machine Learning Pipeline on AWS course is designed to equip learners with a comprehensive understanding of how to create and deploy machine learning models using AWS services. The curriculum is structured into coherent modules that guide students from the basics of machine learning to the complexities of model deployment. In Module 1, participants will grasp the fundamentals of machine learning, exploring various use cases, the types of machine learning, and key concepts. They will also get a thorough overview of the ML pipeline and be introduced to the course projects.Module 2 dives into Amazon SageMaker, providing an introduction and hands-on experience with Jupyter notebooks within the AWS environment. Subsequent modules guide learners through problem formulation, data preprocessing, model training with Amazon SageMaker, model evaluation, and the intricacies of feature engineering and model tuning. The final module, Module 8, covers the crucial aspects of deploying models on Amazon SageMaker, including inference and monitoring, as well as deploying ML at the edge, culminating in a course wrap-up and post-assessment.By the end of the course, participants will have a solid understanding of the ML pipeline on AWS and practical experience that will empower them to tackle real-world machine learning challenges.